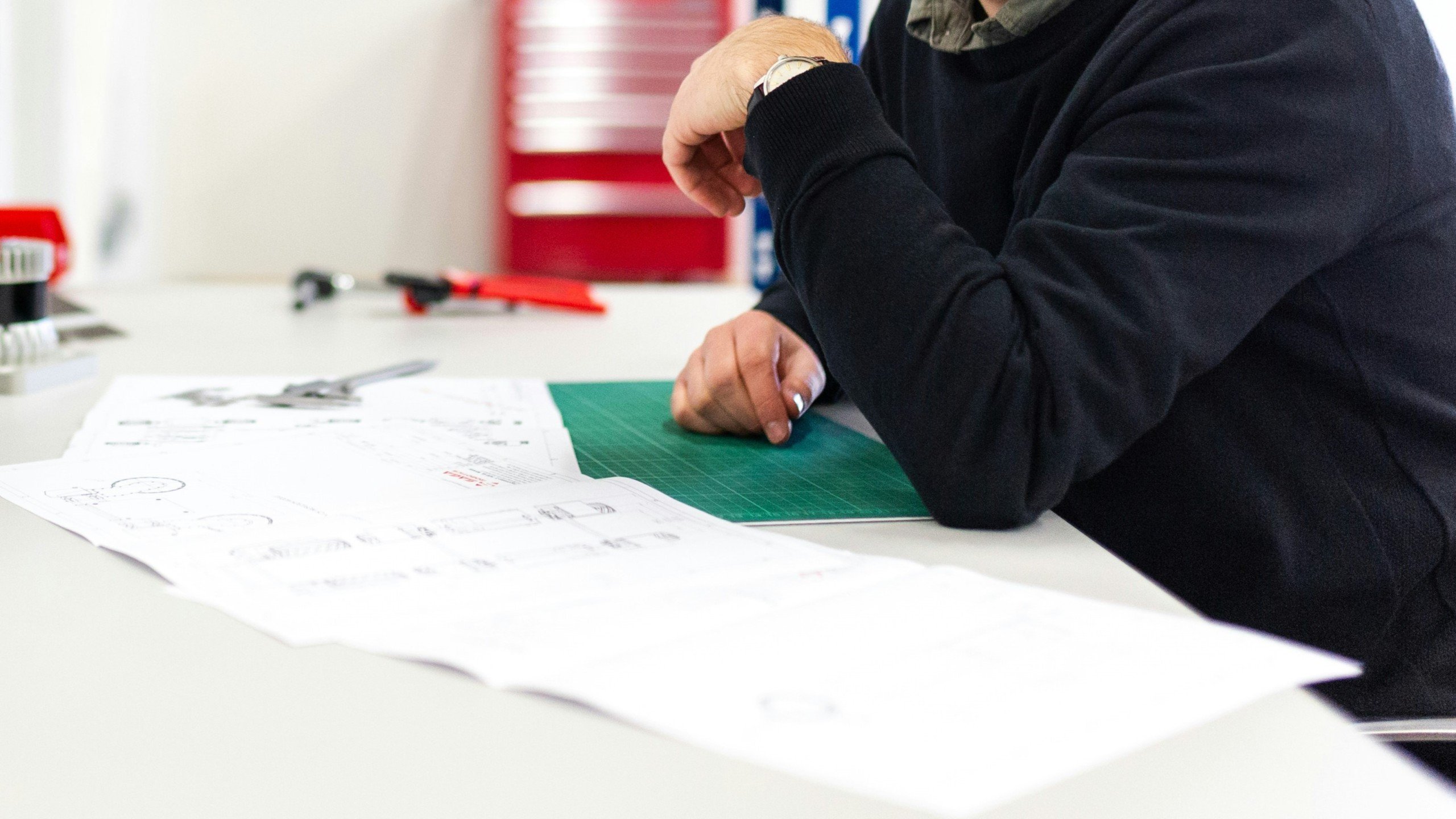
According to a recent Forbes article by Adrian Bridgwater, the deepening penetration of artificial intelligence (AI) across industries highlights both its transformative potential and the challenges in implementation. While AI tools increasingly assist professionals ranging from nurses to engineers, the complexity of enterprise AI deployment underscores the critical importance of data engineering.
Why Data Engineering Matters
The expectation that AI solutions deliver instant magic by merely integrating corporate data is widespread but misguided. As Jim Liddle, Chief Innovation Officer at Nasuni, emphasizes, the largest and least understood obstacle in deploying AI is managing unstructured file data—documents, images, and videos that constitute 90% of all data generated. Unlike structured datasets, these require significant effort to synthesize, curate, and prepare for AI engine consumption.
From Data to Decisions: A Framework for Success
The journey from data chaos to AI-driven clarity involves three critical steps:
- Data Discovery and Organization
- Identifying where unstructured data resides, whether in local storage, cloud environments, or disparate platforms.
- Consolidating this data to eliminate silos and ensure accessibility.
- Curation and Preparation
- Cleaning and organizing data to remove inconsistencies and ensure scalability.
- Normalizing datasets to align with specific AI models and organizational needs.
- Framework Development and Deployment
- Implementing robust data management systems to provide visibility into datasets.
- Establishing mechanisms for ongoing data ingestion, ensuring freshness and relevance for AI models.
Preparing for Increasingly Stringent Regulations
As AI adoption grows, so does regulatory scrutiny. Governments worldwide are introducing new laws to ensure ethical AI usage and data protection. To stay ahead, businesses must understand regional regulations, implement clear internal policies aligned with disclosure agreements with how AI is used, invest in a data governance framework, and conduct regular audits to identify compliance gaps and address them proactively.
Actionable Steps to Get Started with Data Engineering
For businesses looking to embark on their data engineering journey, here are actionable steps to lay a solid foundation:
- Conduct a Data Audit: Identify all sources of data, both structured and unstructured, and assess their quality and relevance.
- Start Small: Implement small-scale AI pilot projects to test and refine your data engineering processes before full-scale deployment.
- Prioritize Data Quality: Focus on cleansing and normalizing data to ensure it is accurate, complete, and consistent.
- Invest in the Right Tools: Use tools that simplify data management tasks, such as ETL (Extract, Transform, Load) platforms, to streamline workflows.
- Build Cross-Functional Teams: Collaborate across departments to ensure data is accessible and actionable for AI applications.
- Monitor and Iterate: Continuously monitor the performance of your data engineering framework and adapt it to changing business needs.
Delivering Measurable Value
As Liddle notes, AI initiatives must demonstrate tangible business outcomes—whether through revenue growth, cost reduction, or operational efficiency. Consider a manufacturing use case: AI-powered inspection tools identify product flaws in real-time, enhancing quality and cutting costs. Similarly, for global marketing firms, AI-driven indexing systems unlock institutional knowledge, enabling faster, more informed creative outputs.
To learn more about the foundational role of data engineering in AI success, read the full Forbes article here.
The Bronson Advantage
At Bronson Consulting, we’re committed to guiding organizations through the complexities of the digital age. Contact us today to discover how we can help you transform your data into a strategic asset.