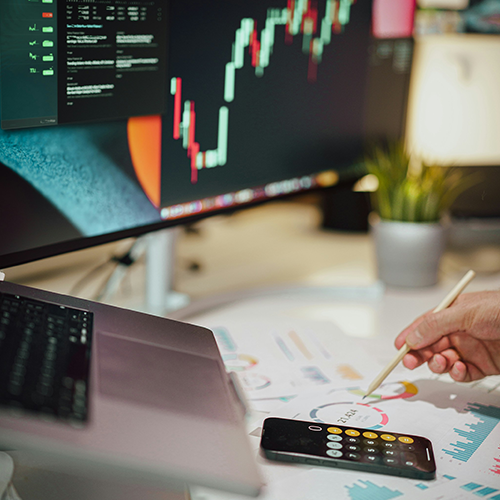
Predictive analytics is the compass that guides organizations through the ever-changing business landscape. By harnessing data and employing sophisticated methodologies, it empowers businesses to anticipate trends, make informed decisions, and stay ahead of the curve. In this article, we dive into the principles and methodologies behind predictive analytics, shedding light on the tools and techniques that enable us to forecast trends with precision.
Principles of Predictive Analytics
At its core, predictive analytics leverages historical data to identify patterns and trends that can be extrapolated into the future. The principles revolve around the idea that past behavior and data can provide valuable insights into what may happen next. Key principles include:
- Data Collection and Preparation: Robust predictive models require clean, comprehensive, and relevant data. The process starts with data collection and encompasses data cleaning, transformation, and feature engineering.
- Model Building: This involves selecting the appropriate predictive model, whether linear regression, decision trees, neural networks, or others, based on the problem at hand.
- Training and Testing: Predictive models are trained on historical data and then tested to ensure they can accurately predict outcomes on unseen data.
- Evaluation: Various metrics, such as Mean Absolute Error (MAE), Mean Squared Error (MSE), or R-squared, are used to evaluate a model’s accuracy.
Methodologies for Predictive Analytics
Predictive analytics encompasses several methodologies, each suited to specific types of data and predictive goals. Three widely used methodologies are:
- Regression Analysis: This method explores relationships between dependent and independent variables. Linear regression, for instance, models a linear relationship between variables, while logistic regression is used for binary outcomes. Regression analysis is valuable for understanding the impact of variables on an outcome.
- Time Series Forecasting: Time series data involves observations collected or recorded at specific time intervals. Time series forecasting methods, such as ARIMA (AutoRegressive Integrated Moving Average) and Exponential Smoothing, analyze historical data patterns to make future predictions.
- Machine Learning Models: Machine learning leverages algorithms to automatically learn patterns from data. Supervised learning algorithms, like decision trees, random forests, and support vector machines, are commonly used for predictive analytics. Unsupervised learning can identify hidden patterns and clusters within data.
Real-World Applications
Predictive analytics finds application across industries:
- Finance: Predictive models assist in stock price forecasting, credit risk assessment, and fraud detection.
- Retail: Inventory management, demand forecasting, and personalized marketing benefit from predictive analytics.
- Healthcare: Predictive models help with disease outbreak prediction, patient readmission risk, and optimizing healthcare resource allocation.
- Manufacturing: Predictive maintenance minimizes equipment downtime, reduces maintenance costs, and enhances production efficiency.
Predictive analytics is the compass that guides organizations through uncertainty, helping them make data-driven decisions and forecast trends accurately. By embracing the principles and methodologies of predictive analytics, businesses across various sectors can unlock the power of data and gain a competitive edge in an ever-evolving world. Whether through regression analysis, time series forecasting, or machine learning models, predictive analytics equips organizations with the insights needed to navigate the complexities of the modern business landscape.
Let Bronson help you unlock the power of your data, one step at a time.