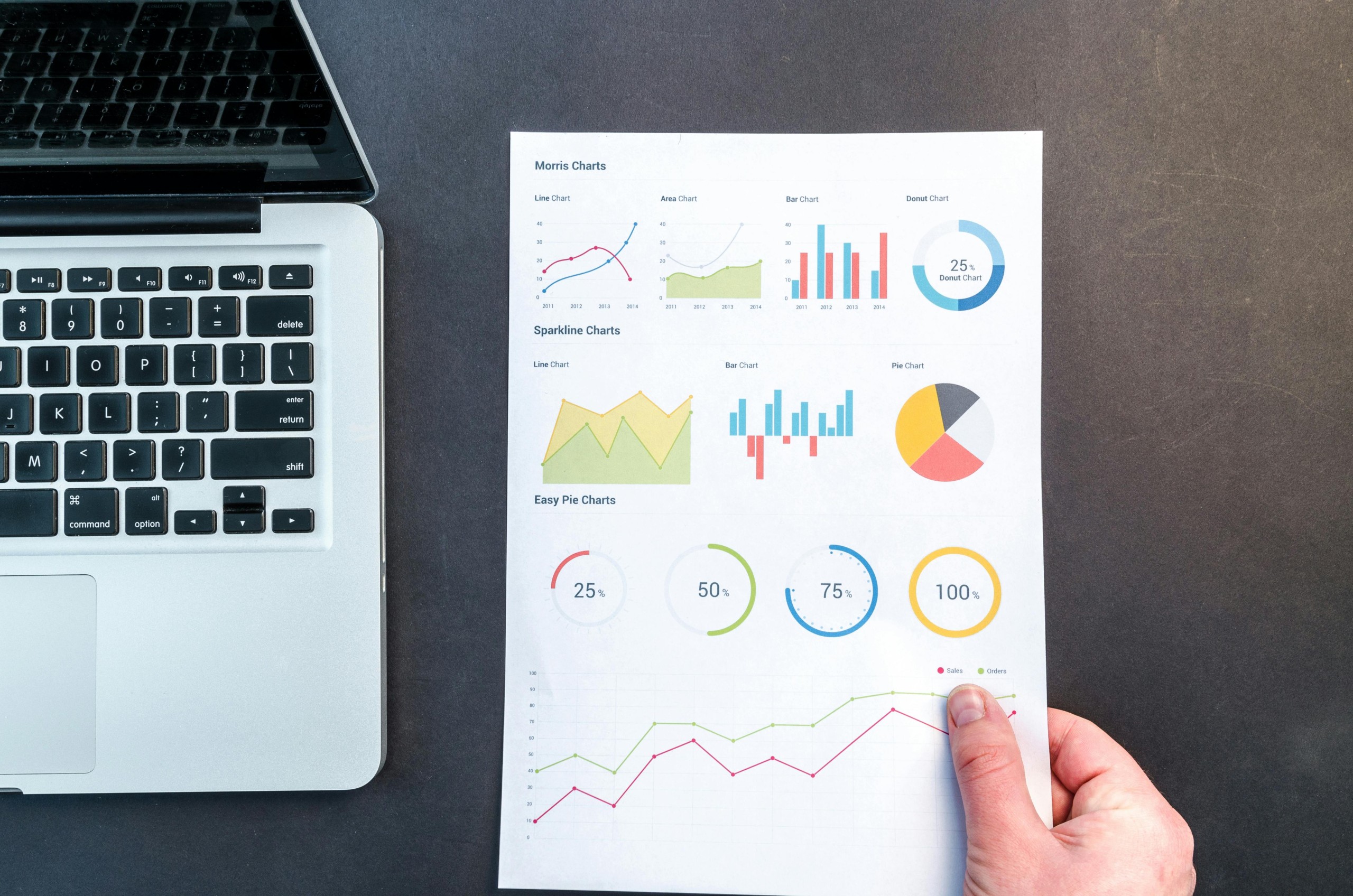
In today’s data-driven world, the immense power of data analytics and artificial intelligence (AI) is transforming industries and shaping human experiences. However, alongside this potential lie intricate ethical challenges that demand our attention. This article dives deep into the ethical considerations surrounding data usage, AI bias, and the responsible handling of data—shedding light on the complexities and offering insights into how we can navigate this evolving landscape.
- Ethical Considerations in Data Usage
The heart of ethical data practices revolves around transparency, consent, and privacy. Organizations must ensure that the data they collect and use is obtained ethically and that individuals are informed about how their data will be utilized. Striking a balance between data-driven innovation and safeguarding personal privacy is a delicate yet essential endeavor. The Cambridge Analytica scandal serves as a stark reminder of the consequences of unethical data usage, emphasizing the need for stringent regulations and ethical guidelines.
- Tackling AI Bias
As AI systems become more prevalent in decision making, addressing biases becomes paramount. AI algorithms can inadvertently perpetuate existing biases present in training data, leading to unfair outcomes. For instance, biased algorithms used in hiring processes can disproportionately disadvantage certain groups. Recognizing and mitigating bias requires continuous monitoring, diverse training data, and algorithmic fairness assessments. Google’s efforts to create guidelines for ethical AI and address bias in AI systems underscore the industry’s commitment to rectifying this issue.
- Responsible Data Handling
The ethical obligation of organizations extends to the responsible handling of data throughout its lifecycle. Data breaches can have severe consequences for individuals and businesses alike. Striving for data security and compliance with regulations such as the General Data Protection Regulation (GDPR) and the California Consumer Privacy Act (CCPA) is crucial. Apple’s emphasis on user privacy, exemplified by features like App Tracking Transparency, sets an example of putting responsible data handling at the forefront of innovation.
- Encouraging Ethical AI Research
The academic and research communities play a significant role in advancing ethical AI. By fostering interdisciplinary collaborations and engaging in discussions around AI ethics, researchers can contribute to the development of frameworks that address ethical concerns. Initiatives like the Fairness, Accountability, and Transparency in Machine Learning (FAT/ML) conference bring together experts to explore methods for making AI systems more transparent and accountable.
As we navigate the intricate landscape of data ethics and AI, it’s imperative to recognize that innovation and responsibility must go hand in hand. Ethical considerations should guide every aspect of data collection, analysis, and application. By championing transparency, fairness, and accountability, we can harness the transformative potential of data and AI while upholding the values that underpin our societies. At Bronson Consulting, we are committed to leading by example in the realm of ethical data practices. Our approach not only ensures compliance but also promotes responsible innovation, safeguarding the interests of individuals and organizations alike. As the data-driven landscape evolves, our commitment remains steadfast, with an unwavering focus on ethical integrity and the creation of a more equitable digital future.