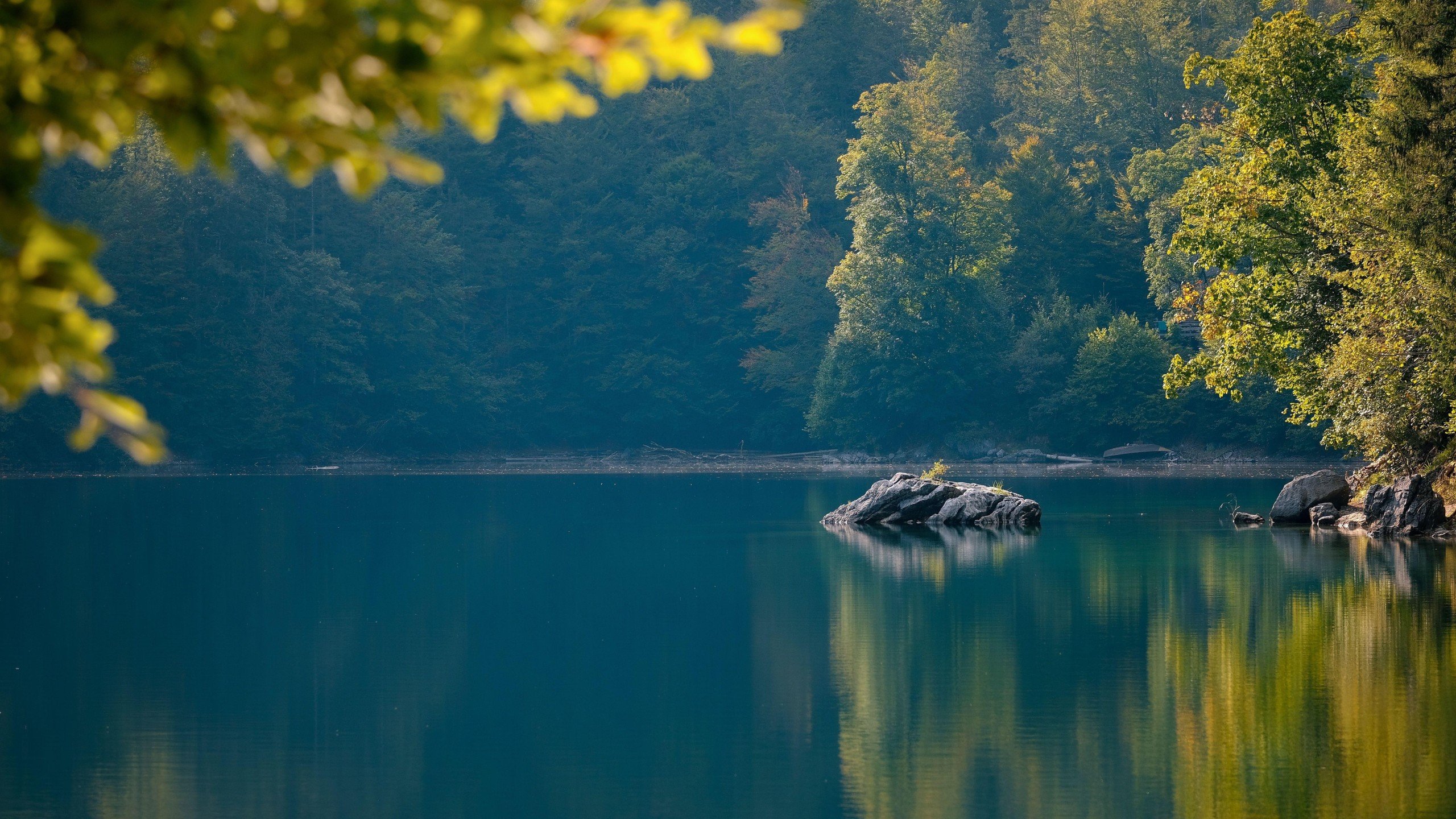
Understanding Data Lakes vs. Data Warehouses: Which is Right for Your Business?
In an era where businesses generate and rely on data more than ever, choosing the right data storage solution is critical. Two of the most popular options are data lakes and data warehouses. While both serve as centralized repositories for storing and analyzing data, they are designed for different purposes and come with distinct advantages. This article will break down the differences, explore their use cases, and help you determine which is best suited for your business needs.
What is a Data Lake?
A data lake is a storage repository that holds vast amounts of raw data in its native format until it’s needed. Think of it as a large reservoir where all types of data—structured, semi-structured, and unstructured—are collected without predefined schemas.
Key Features of Data Lakes:
- Flexibility: Data lakes can store virtually any type of data, including text, images, videos, IoT sensor data, and more.
- Cost-Effectiveness: Because they use low-cost storage options, data lakes are an economical choice for storing large volumes of data.
- Scalability: They can handle massive amounts of data from multiple sources, making them ideal for businesses expecting rapid data growth.
Use Cases for Data Lakes:
- Advanced Analytics and AI: Ideal for data scientists and analysts who need access to raw, unprocessed data for machine learning models and advanced analytics.
- IoT Data: Useful for storing sensor data from connected devices for real-time or batch analysis.
- Data Exploration: Perfect for companies that want to keep data available for future analysis without knowing the exact requirements upfront.
What is a Data Warehouse?
A data warehouse, on the other hand, is a highly structured repository optimized for querying and analysis. Data is cleaned, transformed, and organized into schemas before being stored, ensuring consistency and reliability.
Key Features of Data Warehouses:
- Structured Data: Only structured and semi-structured data is stored, typically in rows and columns.
- Performance Optimization: Designed for fast querying and reporting, making it an excellent choice for business intelligence (BI) tools.
- Data Integrity: Since the data is processed before storage, it is reliable and consistent.
Use Cases for Data Warehouses:
- Business Intelligence (BI): Ideal for generating dashboards and reports that summarize key metrics for decision-makers.
- Historical Data Analysis: Used for analyzing trends over time, such as sales growth or customer retention.
- Regulatory Compliance: Suited for industries like finance or healthcare that require structured, auditable data storage.
Key Differences Between Data Lakes and Data Warehouses
Feature | Data Lake | Data Warehouse |
Data Type | Raw, unstructured, semi-structured, structured   | Structured, semi-structured |
Storage Cost    | Low | Higher |
Processing | Schema-on-read (processed when queried) | Schema-on-write (processed before storage)Â Â Â |
Accessibility | Preferred by data scientists | Preferred by business analysts |
Performance | Slower querying for raw data | Optimized for fast querying |
Use Case | Advanced analytics, ML, AI | BI, reporting, regulatory analysis |
Choosing the Right Solution for Your Business
When deciding between a data lake and a data warehouse, the choice largely depends on your business needs and goals. Here are some scenarios to guide your decision:
- Choose a Data Lake If:
- You need to store diverse data types, including unstructured and semi-structured formats.
- Your business relies on advanced analytics, machine learning, or big data applications.
- You want an economical solution for handling vast amounts of data without immediate analysis needs.
- Choose a Data Warehouse If:
- Your primary focus is on structured, reliable data for reporting and business intelligence.
- You need fast query performance to generate real-time or near-real-time insights.
- You operate in an industry with strict data compliance and governance requirements.
The Hybrid Approach: Data Lakehouses
For businesses with complex data needs, a hybrid solution known as a data lakehouse combines the best of both worlds. A lakehouse enables businesses to store raw data in a data lake while using tools that apply schema and structure as needed, providing the performance benefits of a data warehouse.
This approach is particularly useful for organizations that want to maintain flexibility while ensuring data is accessible and actionable for various stakeholders.
Conclusion
Both data lakes and data warehouses play critical roles in modern data strategies. A data lake offers unmatched flexibility and scalability for handling diverse data types, making it ideal for advanced analytics and innovation. On the other hand, a data warehouse provides structured, reliable data for fast, actionable insights that drive day-to-day business decisions.
By understanding the strengths and limitations of each, you can align your data storage strategy with your organization’s goals. And if you're still unsure, consulting with data experts like Bronson Consulting can help you navigate these options and build a solution tailored to your business needs.
Ready to optimize your data strategy? Contact us to explore how a data lake, data warehouse, or hybrid solution can elevate your business insights.