Chief Data Officer
Data Governance – the 5 “must dos” to implement adaptable data governance
An organization must implement Data Governance to realize it’s data ambitions.
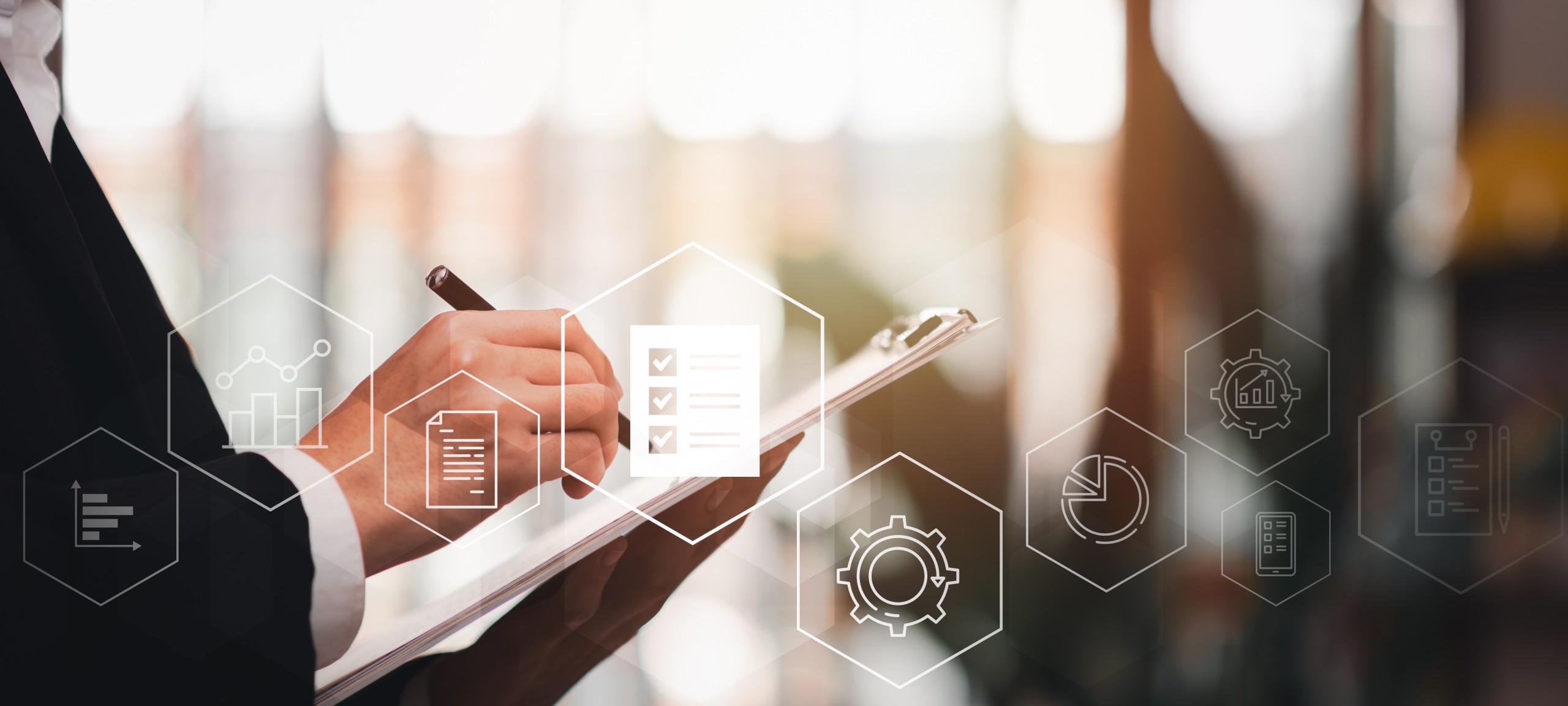
The primary objective of data governance is to ensure that an organization’s data is effectively managed, controlled, and utilized to support its business objectives. The true test of effective data governance is that data is protected and secure and that more and better data is accessible and used for decision support.
Generally, data governance is meant to provide comprehensive framework that encompasses the policies, processes, and controls to ensure that an organization’s data is accurate, available, secure, and utilized effectively. This typically involves identifying roles, responsibilities, and enforcing rules for data management and usage to ensure data quality, integrity, and compliance with relevant policy and regulations. The aim of data governance is to establish accountability, responsibility, and a standardized approach to managing and leveraging data as a valuable organizational asset.
Despite its critical role, many organizations struggle to stand up their data governance practice. As a result, they are often faced with a patchwork and inconsistent approach to data governance. I see 3 main reasons for this:
- The business does not see the compelling reasons that would drive investing time and resources in establishing data governance roles for its data, such as a data steward or data custodians;
- The level of resources required seems out of proportion with the data problem at hand; and
- Organizations are reluctant to take on the change management challenges associated with changing existing job descriptions, training and upskilling.
To overcome these challenges, I recommend organizations take a more practical, adaptable, and risk-based approach to implementing data governance efforts by focusing on 5 areas:
1. Define Your Data Stewardship Model: This is critical. Data stewardship is the cornerstone of effective data governance. It involves defining roles, responsibilities, and processes for managing and safeguarding data. A well-structured enterprise data stewardship model assigns ownership and accountability for data across the organization, ensuring that individuals or teams are responsible for the quality, accuracy, and security of specific datasets. This clarity enhances data integrity, reduces the risk of errors, and fosters a culture of responsibility regarding data assets.
A Typical Data Stewardship Model
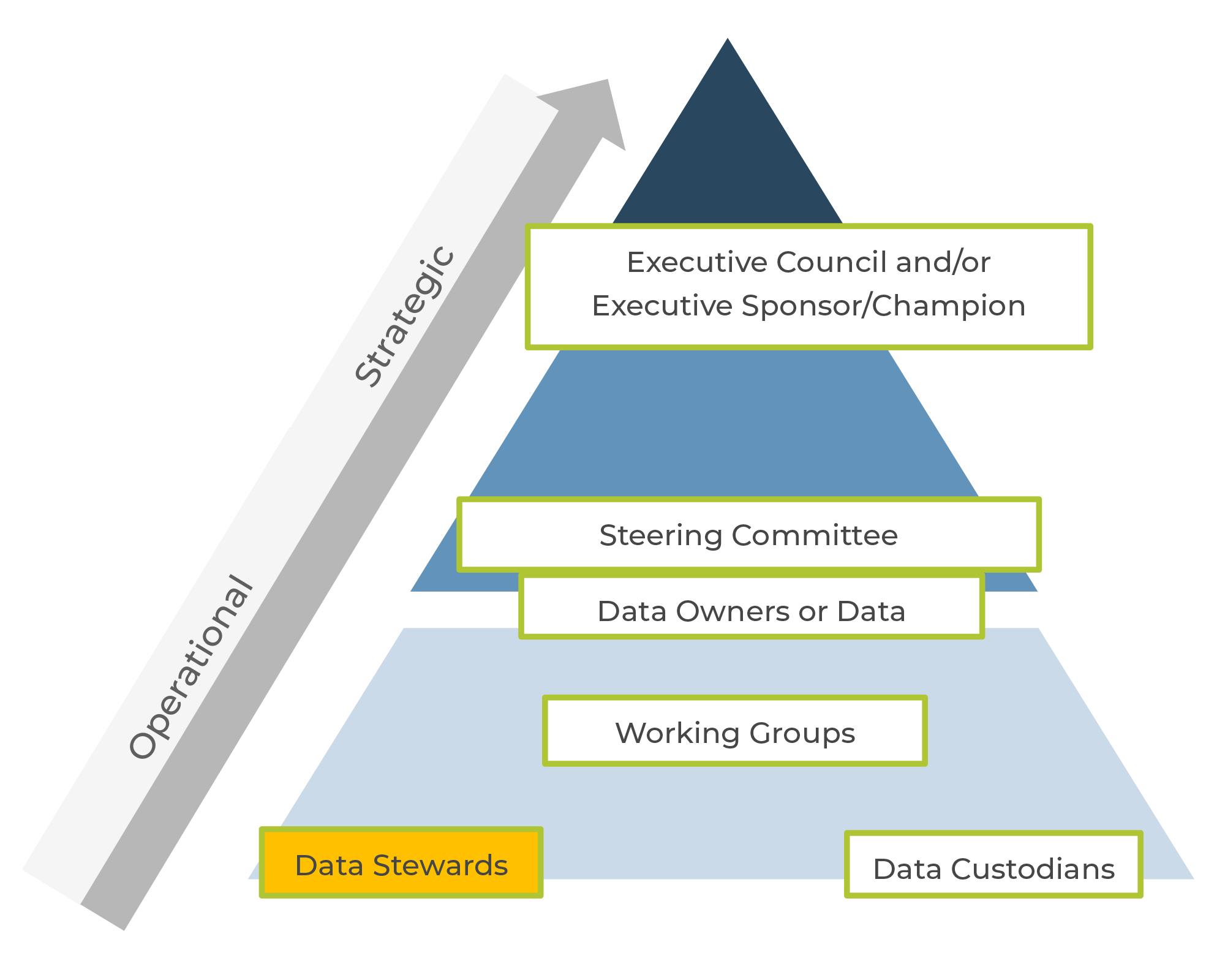
2. Take an Adaptable, Risk-Based Approach: Not all data is created equally. Organizations steward an enormous amount of data, but only a very small percentage is used for decision-support. Understanding which of your data is most critical to achieving business goals helps to identify which data has more value and therefore may need more governance. For example, in some cases, a high level of trust, integrity, and reliability is required to make the best decision possible because of the potential impact. This could include data used to support significant policy changes with nation-wide impacts, data used to support military decisions or actions, or data used by AI tools to decide on eligibility for government programs. Taking a risk-based approach includes 3 considerations:
- To be an effective data steward, organizations need to ensure privacy and security considerations, policies, and tools are in place to protect individual data and the organization. It sometimes takes years to recover from a significant data breach and the damage to organizational reputation can be long lasting;
- Establish a risk-based framework which helps identify the data that is most impactful and critical to the organization. This is the data that likely needs to be governed the most closely; and
- Establish a minimal level of governance (stewardship etc.) for all data and let the business decide on what additional measures need to be put in place based on the results of the risk-based approach.
By focusing efforts on high-risk data, organizations can allocate resources effectively, implement targeted security measures, and ensure compliance with policy and regulations. This approach ensures that data governance efforts are strategic, addressing the most pressing concerns first.
3. Apply to a Real Business Use Case: Data governance should not be a theoretical exercise, but rather a practical, applied discipline. Integrating data governance into a real business use cases demonstrates its tangible benefits. Whether it’s improving the accuracy of individual data, enhancing supply chain visibility, or ensuring regulatory compliance, linking data governance to a specific business scenario provides context and reinforces the importance of data governance, quality, and security. This approach fosters a better understanding of the value that data governance brings to daily operations.
4. Identify the Enterprise Lead for Data Governance: Establishing clear leadership is crucial for the success of any organizational initiative, and data governance is no exception. Many organizations seem to struggle with the need to identify enterprise leadership for the data program. Identifying an enterprise lead for data governance ensures that there is a central authority responsible for overseeing the implementation, enforcement, and continuous improvement of data governance policies. This leader acts as a champion for data governance, fostering a culture of accountability and collaboration across departments.
5. Implement Change Management: Data governance initiatives often entail organizational change. This includes changes and or additions to existing roles, job descriptions, reporting relationships etc. It also requires training the various steward or custodian on their roles and responsibilities. Implementing change management practices helps mitigate resistance, foster acceptance, and promote a smooth transition to the new data governance framework. Effective communication, training programs, and ongoing support are integral components of change management.
The key to the successful implementation of data governance is to be practical and adaptable. Requiring the same level of governance for all data is simply not possible and generates resistance from the business lines who are involved in the implementation. Therefore, organizations should consider a risk-based, flexible approach to ensure their most important data assets are governed effectively.