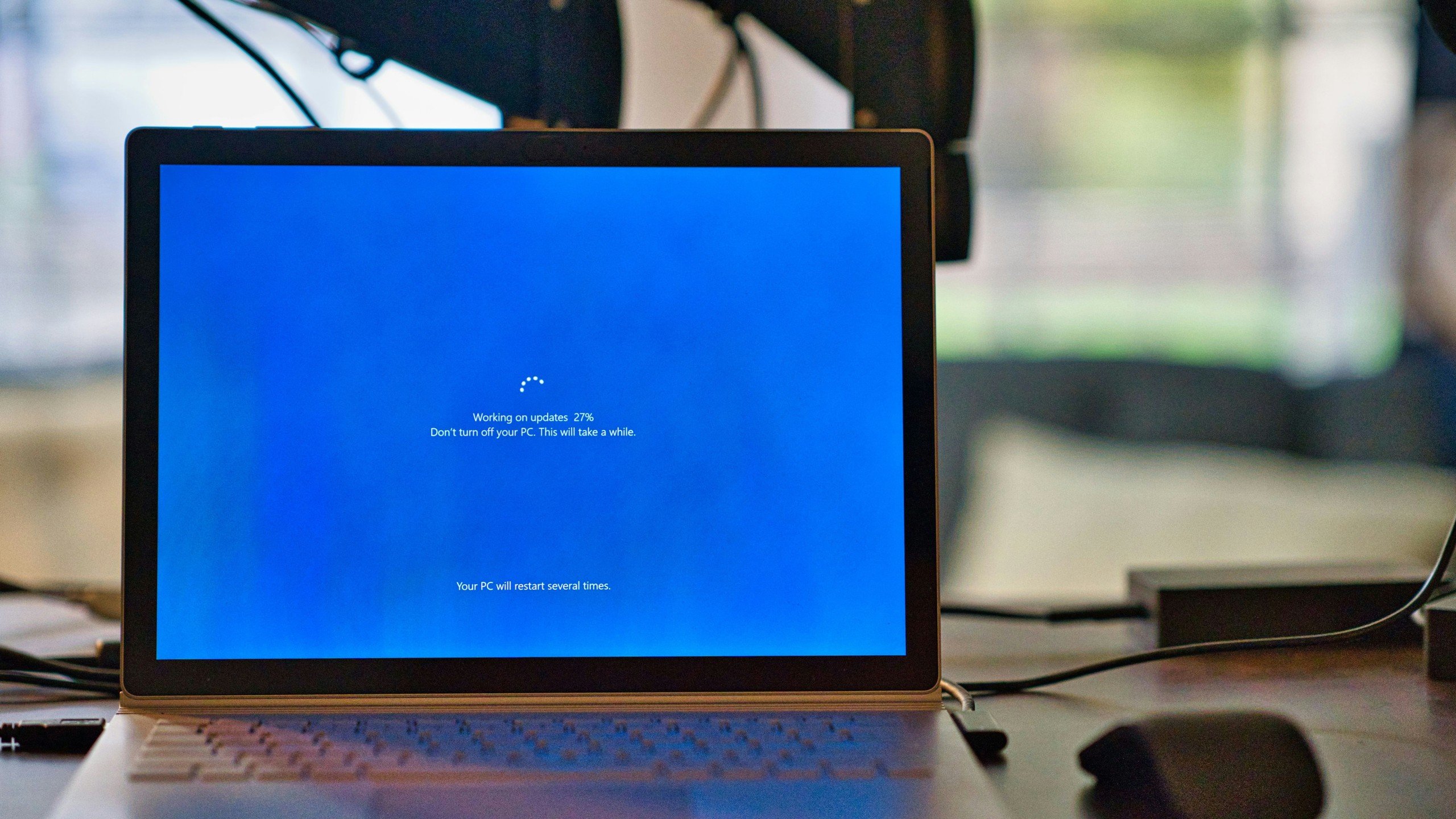
In the age of artificial intelligence (AI), data is often referred to as the new oil—a critical resource powering machine learning models and fueling advancements across industries. However, acquiring high-quality, diverse, and unbiased data for AI training and testing remains a significant challenge. Enter synthetic data: a transformative solution that is reshaping how we think about data generation and utilization.
Synthetic data offers a powerful alternative to real-world data, addressing issues like privacy, scarcity, and bias while unlocking new possibilities for innovation. This article explores the potential of synthetic data in AI training and testing, highlighting its advantages, applications, and impact on the future of AI development.
What is Synthetic Data?
Synthetic data is artificially generated information that mimics the properties and structure of real-world data. It is created using algorithms, simulations, or generative models like GANs (Generative Adversarial Networks). Unlike traditional data collected from actual events or interactions, synthetic data is designed to replicate patterns, distributions, and correlations without directly referencing real-world individuals or entities.
Why Synthetic Data Matters in AI
Maintaining Privacy
Privacy concerns and data regulations like GDPR and CCPA make accessing sensitive information for AI training increasingly complex. Synthetic data circumvents these challenges by generating datasets that retain the statistical properties of the original data without exposing personal or confidential information. This ensures compliance with privacy laws while enabling organizations to leverage valuable insights.
Reducing Bias in AI Models
Real-world data often reflects societal biases, which can lead to skewed and discriminatory AI models. Synthetic data offers a way to counteract these biases by creating balanced datasets that ensure fair representation of all groups. By carefully designing synthetic datasets, developers can address underrepresentation issues and promote equity in AI outcomes.
Overcoming Data Scarcity
In many industries, collecting enough high-quality data is impractical or impossible due to cost, time, or logistical constraints. Synthetic data solves this problem by generating large-scale datasets on demand. For example, in autonomous vehicle training, synthetic environments can simulate countless driving scenarios that would be challenging or unsafe to replicate in real life.
Enhancing Testing and Validation
AI models require rigorous testing across diverse scenarios to ensure reliability and robustness. Synthetic data provides a controlled environment for testing, allowing developers to simulate edge cases, rare events, or unusual behaviors that might not be present in real-world datasets.
Applications of Synthetic Data
- Healthcare: Synthetic data enables access to realistic patient-like datasets without violating privacy, supporting the development of diagnostic algorithms, drug discovery, and predictive analytics.
- Autonomous Vehicles: By simulating diverse driving conditions like traffic, weather, and near-miss scenarios, synthetic data accelerates autonomous vehicle development and prepares models for real-world challenges.
- Finance: Synthetic data allows financial institutions to securely train AI models for fraud detection, risk assessment, and customer behavior analysis by simulating transactions and market conditions.
- Retail and E-Commerce: Retailers leverage synthetic data to optimize demand forecasting, inventory management, and personalized recommendations by replicating shopping behaviors and seasonal trends.
Challenges and Limitations
While synthetic data offers immense potential, it also comes with notable challenges. Maintaining realism is critical, as synthetic datasets must accurately represent real-world conditions to ensure effectiveness. Poorly designed data can lead to overfitting or unrealistic model behavior, undermining reliability. Generating high-quality synthetic data is often complex and resource-intensive, requiring advanced tools like GANs and simulation software, along with significant expertise. Additionally, businesses and regulators may question the reliability of AI models trained on synthetic data. Ensuring that synthetic datasets align closely with real-world data distributions is essential for building trust and validating their outcomes.
The Future of Synthetic Data
As AI continues to evolve, synthetic data will play an increasingly critical role in shaping its development. Advances in generative models, such as diffusion models and improved GANs, promise to make synthetic data even more realistic and versatile. Additionally, the growing emphasis on ethical AI development positions synthetic data as a cornerstone for creating fairer and more inclusive systems.
Organizations adopting synthetic data are not just addressing current challenges—they are also paving the way for a more innovative and responsible AI landscape. By prioritizing privacy, reducing biases, and overcoming data limitations, synthetic data empowers businesses to unlock the full potential of AI while maintaining ethical and practical standards.
Conclusion
Synthetic data is more than just a technological innovation—it is a paradigm shift in how we approach data generation, privacy, and AI development. From training machine learning models to testing complex scenarios, its applications are as diverse as they are transformative.
At Bronson Consulting, we’re committed to helping organizations navigate the opportunities and challenges of synthetic data, ensuring they stay ahead in the rapidly evolving AI landscape.